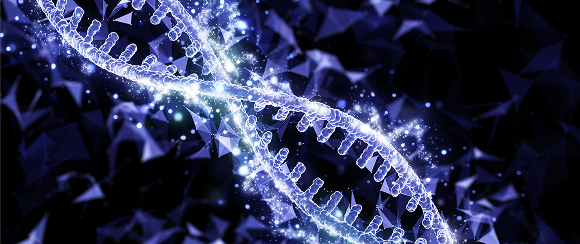
LSM vs LLMs: Smarter mRNA Sequence Design with Transformer Networks
LSM vs LLMs: Smarter mRNA Sequence Design with Transformer Networks
Since Covid-19, mRNA based therapeutics have emerged as effective alternatives and hence design of mRNA sequences is an urgent and essential step. Smart design AI models are essential since traditional codon optimization algorithms will take years to optimize from a huge number of sequence possibilities. Also, it is very much essential to consider structural information along with biological sequences unlike general language sequences. Recently, there is much attention towards transformer networks that have emerged as powerful tools in biological sequence modeling such as from predicting 3' UTRs to optimizing mRNA stability, transformers are reshaping how we design genetic constructs. But when it comes to choosing Large Sequence Models (LSM) vs Large Language Models (LLMs) for mRNA sequence design, the differences are critical—and practical.
Same Architecture, Different Mindset
At first glance, LLMs and domain-specific LSM models may look alike—they both use transformer blocks, attention mechanisms, and tokenized sequences. But what separates them is purpose and precision. LLMs like GPT or BERT are designed for natural language, trained on vast corpora of human text. In contrast, domain-specific LSM for mRNA design are built from ground truth experimental small biological data sets to understand underlying biology for smart design, not a text summary.
Why Domain-Specific LSM Models
Efficient Learning from Small Data
Unlike LLMs, which need billions of tokens, specialized LSM models learn from curated biological datasets. Codon usage, secondary structure, and ribosome profiling data—these are the real “features” that matter to understand biology.
Built-in Biological Intelligence
mRNA sequence design isn’t just about string generation. It’s about preserving folding patterns, avoiding immunogenic motifs, and enhancing translational efficiency. LSM models can integrate these constraints natively through custom loss functions, token schemes, or structure-aware attention.
Interpretable and Purpose-Driven
In contrast to LLMs, domain-specific LSM models offer insights—highlighting motifs, base-pairing tendencies, or sequence features which are interpretable that biologists can trust.
Lower Resource, Higher Relevance
Fine-tuning LLMs requires massive infrastructure. A well-trained deep learning LSM model for mRNA design can run on a standard GPU—making it practical for biotech startups, academic labs, and scalable pipelines.
The Bottom Line
LLMs are incredible for language—but mRNA isn't language. It's biology, with structure, function, and purpose. For real-world applications like mRNA therapeutics, vaccine design, or gene regulation studies, domain-specific deep learning based LSM models powered by transformers are the smarter, leaner, and biologically aligned choice.
- Patents (2)
- Publications (list 5)

What is Lorem Ipsum?
Lorem ipsum dolor sit amet, consectetur adipiscing elit. Suspendisse varius enim in eros elementum tristique. Duis cursus, mi quis viverra ornare, eros dolor interdum nulla, ut commodo diam libero vitae erat. Aenean faucibus nibh et justo cursus id rutrum lorem imperdiet. Nunc ut sem vitae risus tristique posuere.
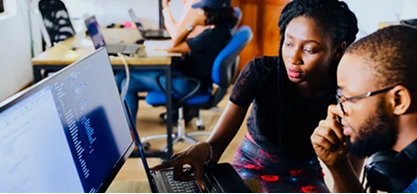
What is Lorem Ipsum?
Lorem ipsum dolor sit amet, consectetur adipiscing elit. Suspendisse varius enim in eros elementum tristique. Duis cursus, mi quis viverra ornare, eros dolor interdum nulla, ut commodo diam libero vitae erat. Aenean faucibus nibh et justo cursus id rutrum lorem imperdiet. Nunc ut sem vitae risus tristique posuere.
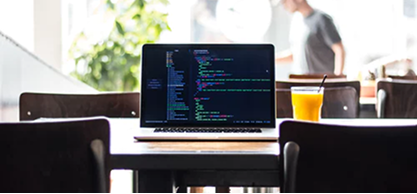
What is Lorem Ipsum?
Lorem ipsum dolor sit amet, consectetur adipiscing elit. Suspendisse varius enim in eros elementum tristique. Duis cursus, mi quis viverra ornare, eros dolor interdum nulla, ut commodo diam libero vitae erat. Aenean faucibus nibh et justo cursus id rutrum lorem imperdiet. Nunc ut sem vitae risus tristique posuere.